The Boeva Lab develops advanced computational methods at the intersection of machine learning and cancer biology. Our goal is to extract actionable insights from high-dimensional molecular data to better understand cancer mechanisms, from genetic regulation to treatment response. We collaborate across disciplines to drive translational research that can ultimately benefit patients through personalized oncology.
Research Mission
Key Research Directions
1. Multiscale Modeling of DNA Regulatory Elements
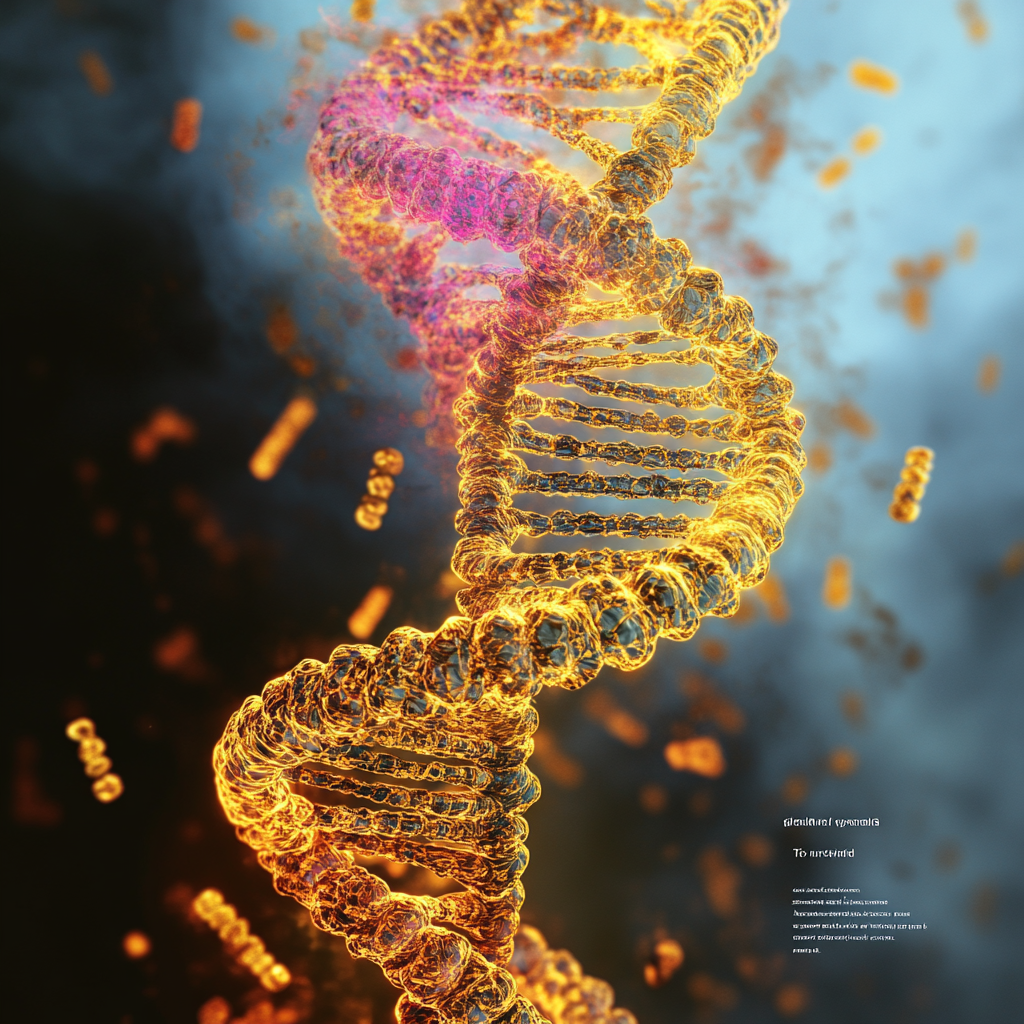
We build computational models to understand how non-coding mutations—representing over 90% of somatic mutations in cancer—impact gene regulation.
This work is supported by the SDSC Collaborative Data Science Project and aligns with our broader goal to model genome-wide variant effects and train cancer-specific foundation models on multiomic data.
Our recent work includes:
- Evaluation of advanced deep learning architectures (e.g., ConvNeXt blocks) to predict high-resolution chromatin accessibility from DNA sequence; Developement of the ASAP framework to assess effects of non-coding variants [BioRxiv].
- A breakthrough attention-based deep ensemble model, UniversalEPI, to link non-coding variants with 3D chromatin structure changes and their downstream transcriptional consequences [BioRxiv].
Our ASAP and UniversalEPI models provide accurate predictions of chromatin changes induced by non-coding variants. The two models can be combined to offer alternatives to experimental assays and advance our understanding of regulatory mutation effects in organism development and diseases, such as cancer. Additionally, the confidence estimates (aleatoric and epistemic uncertainty) of UniversalEPI allow for the detection of differential chromatin folding across conditions in a cell-type-specific manner.
Our published work includes:
- Development of a Latent Dirichlet Allocation (LDA) model to predict the tissue-specific effects of non-coding mutations based on epigenetic context [ AJHJ, 2018].
- Co-development of CHROMATIX, a framework for computing many-body chromatin interactions from deconvolved single-cell data [ Genome Biology, 2020].
2. Molecular Signal Deconvolution
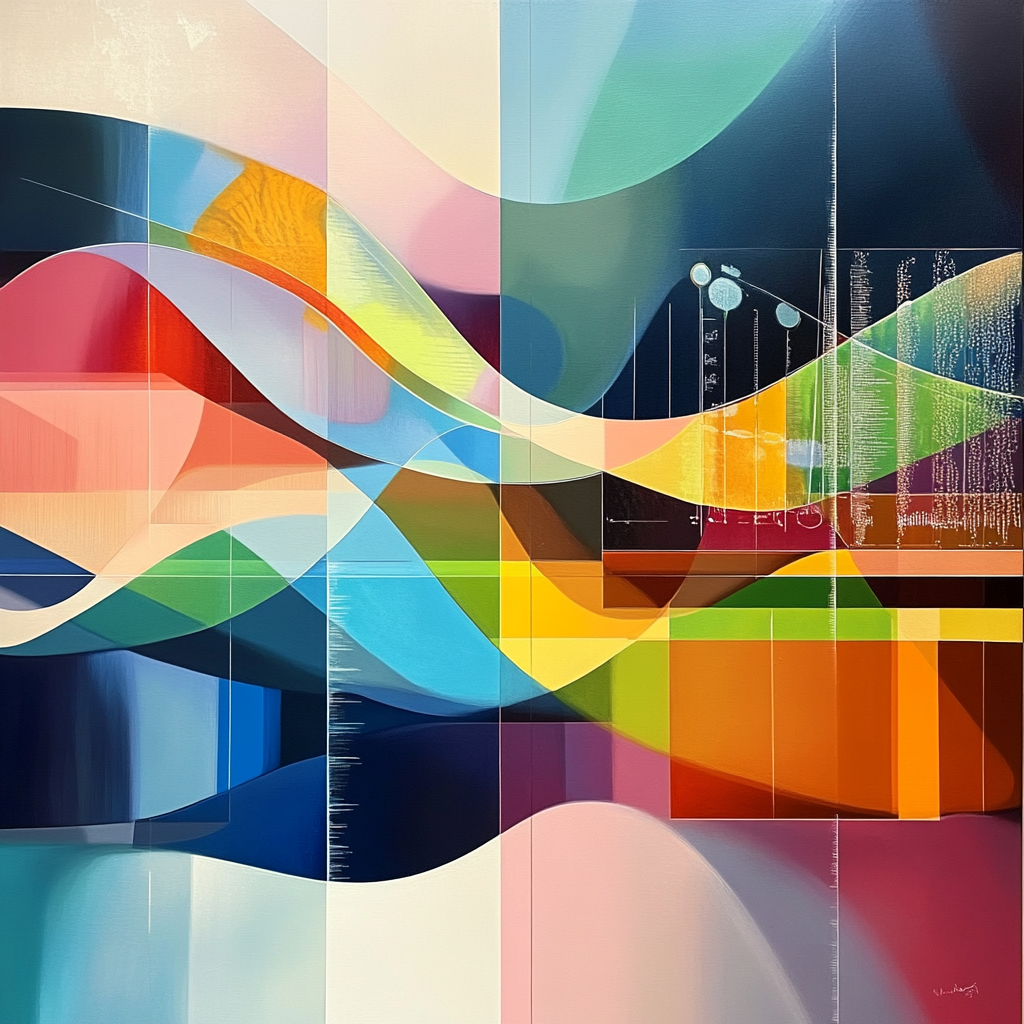
Tumor samples are inherently heterogeneous, composed of malignant cells intermixed with non-malignant populations (e.g., immune or stromal). Bulk omics data lacks the resolution to separate these sources directly, yet it remains the most accessible form of data in clinical contexts. Our lab develops computational approaches to extract cancer-cell-specific molecular signals from such mixed datasets.
- We introduced a deconvolution approach, CDState, based on constrained non-negative matrix factorization with cosine distance regularization to recover cancer-specific transcriptomic profiles [ BioRxiv]. CDState was applied to 33 cancer types to identify somatic genomic variants associated with elevated transcriptional tumor heterogeneity — highlighting the potential pan-cancer role of mutations in the TP53 gene in affecting the distribution of tumor cell states.
- We analyzed DNA hypermethylation across 19 cancer types using deconvolved signals, identifying epigenetic biomarkers and regulatory disruptions in pre-cancerous lesions [Briefings in Bioinformatics, 2022].
These findings suggest new avenues for identifying transcriptional states and studying the link between transcriptional plasticity and treatment resistance. Our ongoing efforts explore how machine learning models trained on single-cell data (e.g., transcriptional foundation models) can be adapted to infer hidden structure in bulk tumor signals. This line of work is supported by the LOOP Zurich platform and SNF grants.
3. Multiomics Survival Models
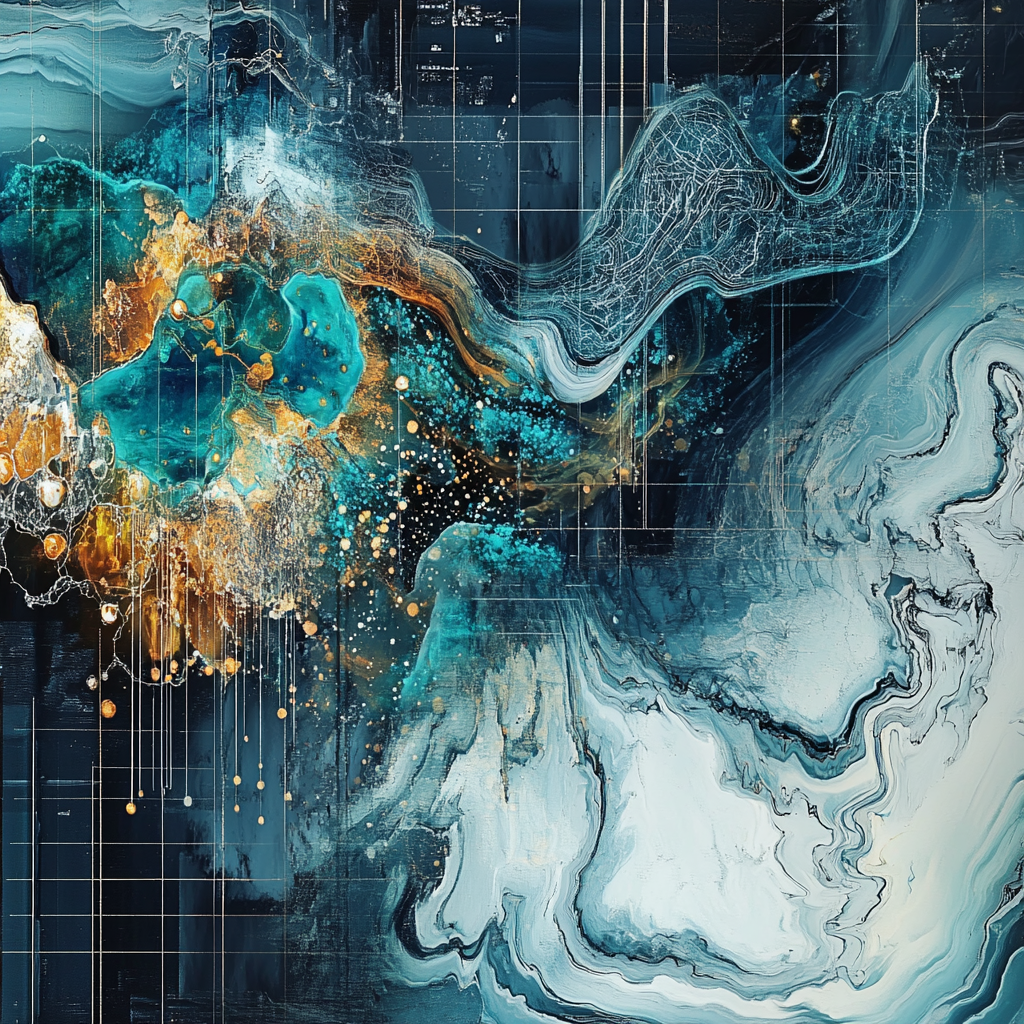
Patient survival and treatment response are influenced by complex, multi-layered molecular signals. We develop interpretable and robust models that integrate genomic, epigenetic, transcriptomic, and imaging data to improve clinical outcome prediction in cancer.
- We proposed a novel knowledge distillation framework that produces accurate yet interpretable survival models from high-dimensional omics data [Bioinformatics, 2024].
- We benchmarked the robustness of multi-modal survival models, showing that all current architectures fail under realistic data noise scenarios [Cell Reports Methods, 2023].
- We developed SurvBoard, the first comprehensive benchmarking framework for survival prediction from multiomics data. It enables standardized evaluation of model accuracy, robustness, and interpretability across cancer types [BioRxiv].
Our tools establish community standards for evaluating single- and multiomics survival prediction models. We are now exploring the use of foundation model-derived embeddings to improve generalization, calibration, and interpretability of survival models in noisy clinical data settings.
This direction is supported by the SNF Sinergia grant and aligns with our broader goal to deliver AI-driven clinical tools with real-world translational value in oncology.
4. AI-Driven Biomarker Discovery
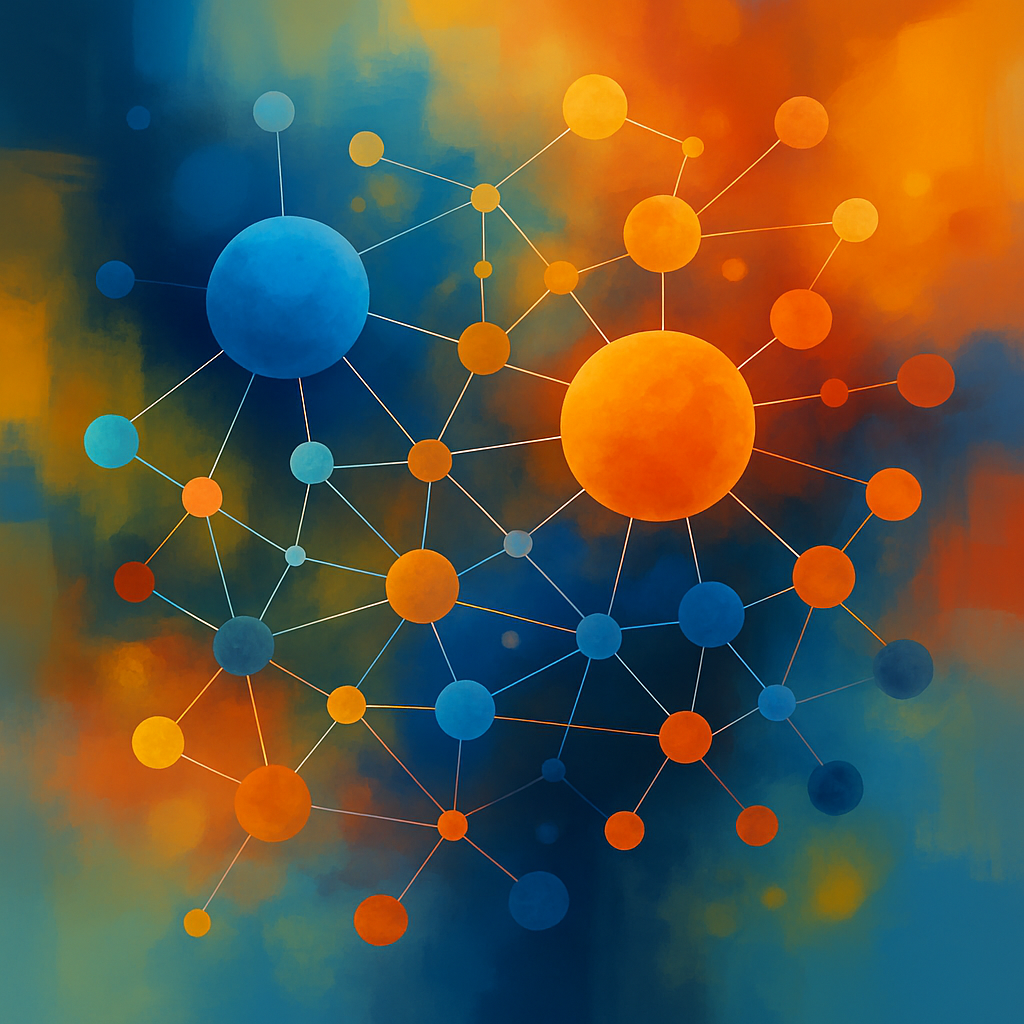
Modern spatial and single-cell omics technologies produce high-dimensional data across transcriptomics, epigenetics, and imaging. We design computational frameworks that integrate these data types to uncover robust biomarkers for cancer diagnosis, classification, and treatment response prediction.
- We introduced CancerFoundation, one of the first cancer-specific foundation models, trained to generate cell embeddings suitable for zero-shot data integration and drug response prediction [BioRxiv].
- We benchmarked multiple self-supervised learning methods on single-cell data, evaluating their ability to correct batch effects and predict missing modalities across datasets [ICML proceedings, 2025].
- In collaboration with Yale, we developed cell-graph learning framework for analyzing spatial omics data and identifying morphologically defined neighborhoods associated with treatment response [BioRxiv].
These models allow us to identify biomarkers from tissue images, spatial transcriptomics, and single-cell data in a biologically interpretable and computationally scalable manner. We are also exploring optimal transport-based tracking of cells in tissue sections and tumor progression trajectories.
This research is supported by the Swiss AI and aims to build multimodal AI systems for precision oncology.
Research Areas and Cancer Types
We investigate a range of cancers including esophageal adenocarcinoma, mesothelioma, lung cancer, neuroblastoma, adrenocortical carcinoma, Ewing sarcoma, and lymphoma. We are open to collaborations across cancer types and domains where computational genomics can add value.
Resources
- Complete list of publications
- Complete list of developed software
- We offer several internship projects to bachelor and master’s students. Please check this page for more information!